In the rapidly evolving landscape of artificial intelligence, the debate between the capabilities of RL-trained reasoners and traditional large language models (LLMs) continues to gain traction. A recent tweet highlighted a critical observation: on open-ended questions, reasoners perform no better than traditional LLMs. This raises an intriguing question: can you distinguish between a model trained with reinforcement learning (RL) for reasoning and a traditional LLM thinking step-by-step?
Understanding the Capabilities of LLMs
Large language models have been at the forefront of AI advancements, with companies like OpenAI, Google, and Meta leading the charge. These models are designed to process and generate human-like text by leveraging vast amounts of training data. However, their ability to perform genuine logical reasoning has been a topic of debate. According to a recent article by Apple, current LLMs cannot perform genuine logical reasoning; they replicate reasoning steps from their training data.
RL-Trained Reasoners: A Step Forward?
Reinforcement learning (RL) has been proposed as a method to enhance the reasoning capabilities of AI models. The idea is to train models to reason through complex problems by breaking them down into smaller steps, a technique known as ‘chain-of-thought’ reasoning. OpenAI’s recent launch of the ‘Strawberry’ series, including the o1 and o1-mini models, aims to leverage this approach. These models are designed to excel in science, coding, and math tasks by spending more time processing answers.
Performance Comparison: RL-Trained Reasoners vs. Traditional LLMs
Despite the advancements, the performance of RL-trained reasoners on open-ended questions remains comparable to traditional LLMs. This observation aligns with the sentiment expressed in the tweet. The challenge lies in distinguishing between a model trained with RL for reasoning and a traditional LLM thinking step-by-step. As noted by Paras Chopra, reasoning is knowing an algorithm to solve a problem, not solving all of it in your head.
Applications and Limitations
Both RL-trained reasoners and traditional LLMs have their unique applications and limitations. For instance, LLMs have been successfully integrated into home robotics to help robots recover from errors without human intervention. MIT researchers have developed a method for robots to self-correct errors using LLMs and imitation learning. This approach enables robots to adjust to environmental variations by breaking down tasks into subtasks and utilizing LLMs for natural language understanding and replanning.
However, the limitations of LLMs become evident in specific tasks. A study revealed that including evidence in questions can confuse models like ChatGPT, lowering their accuracy. This highlights the need for further research to improve the robustness and reliability of these models.
Future Directions and Ethical Considerations
As AI continues to evolve, the focus on enhancing reasoning capabilities will likely intensify. Companies like OpenAI are already exploring new techniques to improve the performance of their models. However, ethical considerations must be addressed to ensure responsible AI development. The potential for misuse of LLMs, such as generating harmful or biased instructions, underscores the need for transparency and accountability in AI research.
In conclusion, while RL-trained reasoners offer promising advancements, their performance on open-ended questions remains on par with traditional LLMs. The ongoing research and development in this field will undoubtedly shape the future of AI reasoning capabilities.
Related Articles
- The Next Leap in AI: Quantum Machine Learning on Quantum Computers
- Exploring the Inner Workings of Large Language Models (LLMs)
- Human Creativity in the Age of LLMs
- Navigating the Complexities of LLM Development: From Demos to Production
- Rethinking LLM Memorization
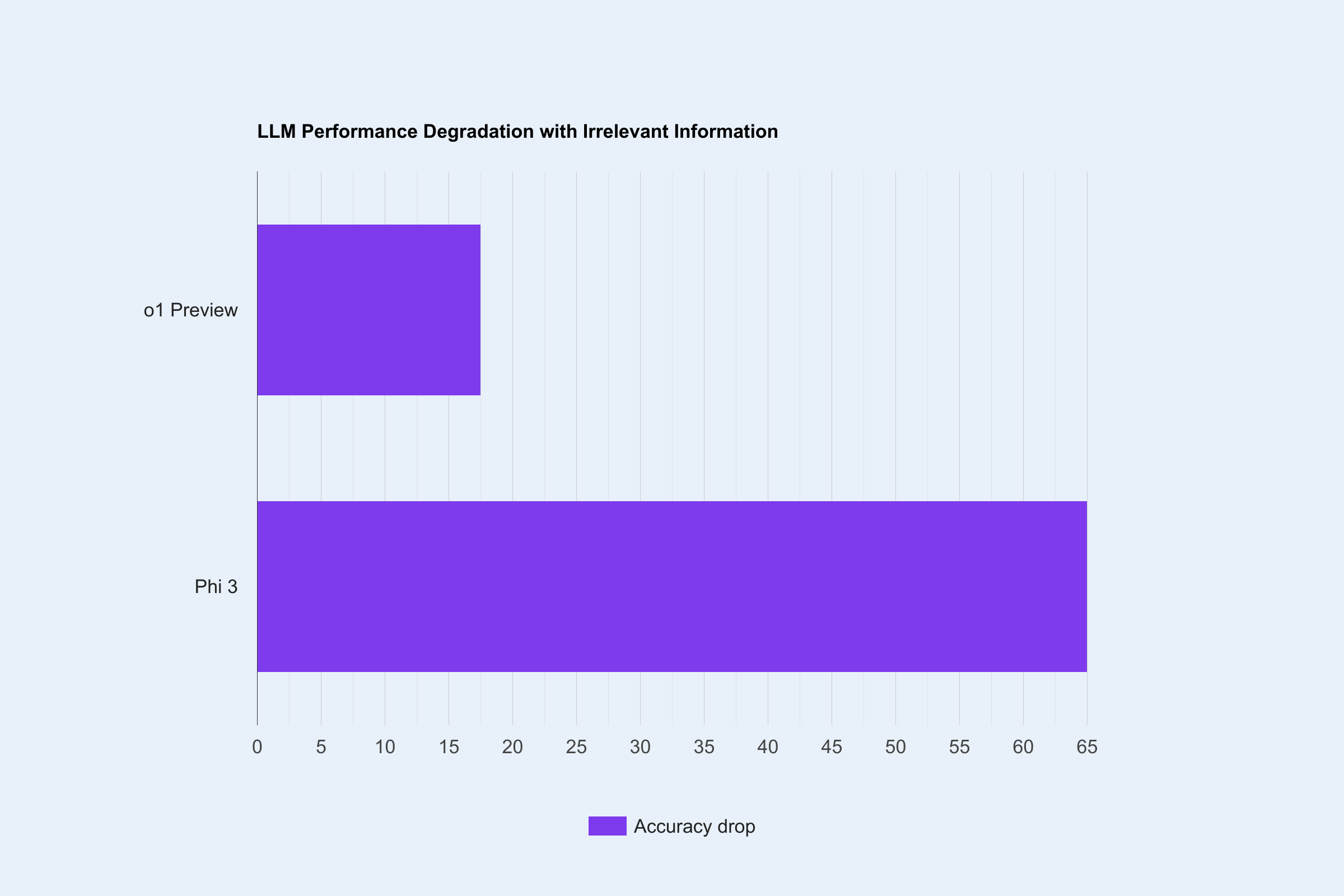
Looking for Travel Inspiration?
Explore Textify’s AI membership
Need a Chart? Explore the world’s largest Charts database