AI Agents: Revolutionizing Data Curation
The advent of AI agents capable of transforming raw documents into rich training examples without human intervention marks a significant milestone in the field of artificial intelligence. A recent paper from Microsoft highlights the potential of these autonomous AI agents in addressing the original problem of creating high-quality synthetic data for training large language models (LLMs), which traditionally requires significant human effort in curation.
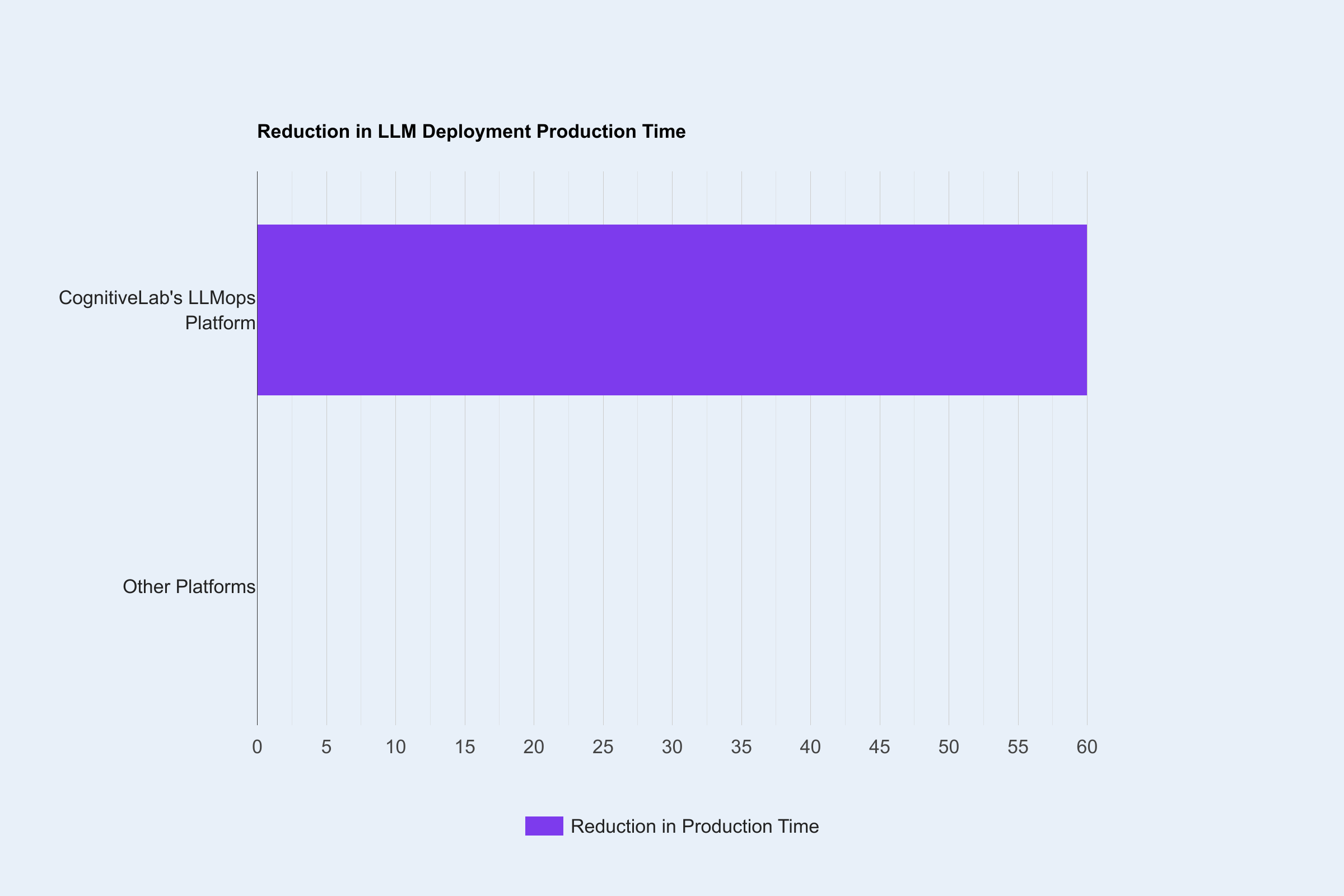
HerculesAI: Pioneering AI-Powered Automation
HerculesAI, formerly known as Zero Systems, has been at the forefront of working with large language models long before it became mainstream. Their AI-powered automation platform focuses on intelligent data extraction, transformation, and verification, particularly valuable for regulated industries like legal, insurance, and financial services. The platform’s unique selling proposition lies in its multi-agent system that automates decision-making processes, ensuring data verification against the source of truth. This approach is crucial for industries dealing with large volumes of data and complex compliance requirements. HerculesAI has been working with LLMs long before it was cool.
Anthropic’s Focus on AI Safety and Ethics
Anthropic, another key player in the AI field, emphasizes building safe and reliable AI systems. Their research highlights vulnerabilities in current LLM technology, where persistent questioning can bypass safety guardrails, leading to the generation of harmful content. This raises concerns about the safety and reliability of LLMs, potentially impacting their adoption and development. The findings have significant implications for the development and deployment of LLMs in various applications, such as chatbots, content creation, and customer service. Anthropic found a trick to get AI to give you answers it’s not supposed to.
Apple’s Responsible Approach to AI Training
Apple has taken a responsible approach to training its Apple Intelligence models, sourcing training data in a way that aligns with their core values and responsible AI principles. The training data for Apple Foundation Models (AFM) includes publicly available web data and licensed data from undisclosed publishers. Apple emphasizes the importance of ethical considerations in AI training, ensuring transparency and avoiding legal scrutiny. Apple says it took a responsible approach to training its Apple Intelligence models.
Meta’s Open AI Model
Meta has released its biggest open AI model yet, reflecting the growing interest in generative AI and models with larger context windows. This model aims to make LLMs more accessible and easier to manage for enterprises, potentially impacting various industries. The focus on making LLMs practical for enterprise use cases highlights the trend of enterprises adopting LLMs and generative AI. Meta releases its biggest open AI model yet.
AI21 Labs’ Jamba Model
AI21 Labs has introduced Jamba, a text-generating and analyzing AI model with a large context window. This model uses a combination of transformer and state space model (SSM) architectures for efficient processing of long contexts, enabling better comprehension and generation of text. Jamba can handle up to 140,000 tokens on a single GPU, delivering three times the throughput on long contexts compared to similar-sized transformer models. AI21 Labs’ new AI model can handle more context than most.
Related Articles
- 5 ways to implement AI into your business now
- Top 7 legal AI tools for law practitioners
- Launching Deep Lex: Where law meets AI
- Claude 3.5 Sonnet: A leap forward in AI document analysis
- Google’s new AI-powered documentation tool for healthcare
Looking for Travel Inspiration?
Explore Textify’s AI membership
Need a Chart? Explore the world’s largest Charts database