Vector Databases: A Misfit for AI Applications
The rise of AI applications has brought vector databases into the limelight. Companies like Qdrant and Marqo have been at the forefront, offering vector databases for storing and processing data in the form of vector embeddings. These databases enable efficient similarity search and retrieval for AI/ML applications. However, recent discussions suggest that vector databases might be the wrong abstraction for AI apps. According to a recent tweet, treating embeddings as derived data, not independent, can eliminate sync nightmares. This approach can simplify management and eliminate the need for juggling multiple databases.
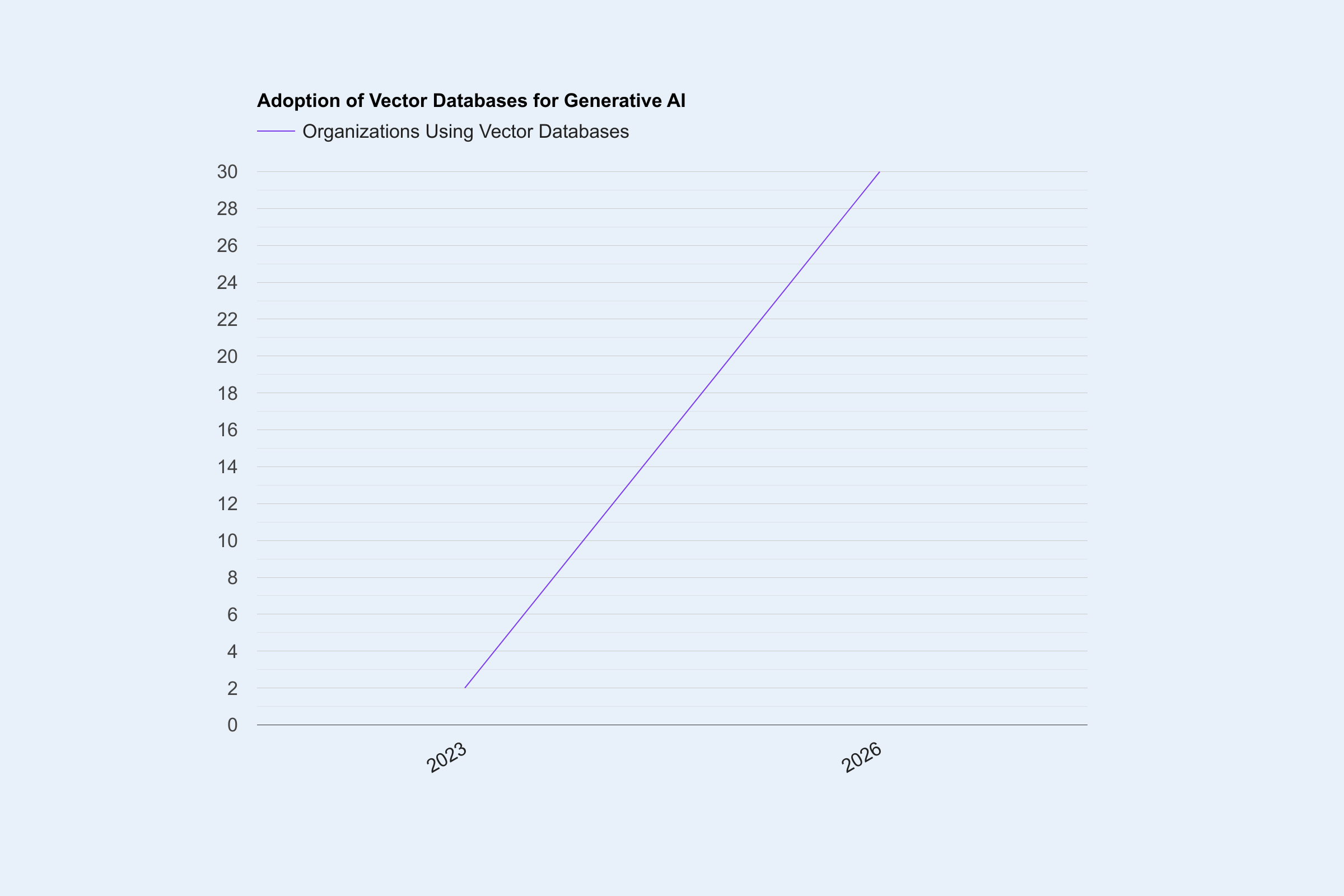
The Future with Vectorizers in PostgreSQL
The tweet also highlights the potential of vectorizers in PostgreSQL. By integrating vectorizers within PostgreSQL, developers can benefit from automatic updates and simplified management. This approach eliminates the need for multiple databases, making it a more efficient solution for AI applications. The integration of vectorizers in PostgreSQL represents a significant shift towards more streamlined and manageable AI data solutions.
The Rise and Challenges of Vector Databases
Vector databases have seen a surge in popularity, especially with the growing adoption of AI/ML. Companies like Pinecone have introduced serverless architectures for vector databases, offering significant cost reductions and seamless integration with various AI and backend services. Despite these advancements, the fundamental abstraction of vector databases for AI applications remains a topic of debate. The complexity of managing multiple databases and ensuring data synchronization poses significant challenges.
Case Studies and Market Trends
Companies like Qdrant have raised substantial funding to enhance their vector database offerings. Qdrant’s open-source vector database and search engine provide efficient binary quantization compression technology, significantly reducing memory consumption and enhancing retrieval speeds. Despite these advancements, the debate on the suitability of vector databases for AI applications continues. The market trends indicate a growing interest in more integrated and simplified solutions, such as vectorizers in PostgreSQL.
Ethical Considerations and Future Directions
As with any AI technology, ethical considerations are paramount. The potential bias in data and algorithms used for vector embeddings can lead to unfair or discriminatory outcomes. Companies must address these concerns while developing and deploying AI applications. The future direction seems to be leaning towards more integrated solutions like vectorizers in PostgreSQL, which promise automatic updates, simplified management, and a unified database approach.
Related Articles
- Vectorizer AI
- Revolutionizing 3D Creation with AI
- Transform Your Ideas into Stunning Images with Segmind Vega
- Redefining Data Intelligence at Internet Scale
- How AI is Revolutionizing B2B Data Analytics
Looking for Travel Inspiration?
Explore Textify’s AI membership
Need a Chart? Explore the world’s largest Charts database