In the ever-evolving world of technology, the terms “AI” and “Machine Learning” often intertwine. As we venture further into this digital age, understanding the distinctions and overlaps between these two domains becomes increasingly essential.
This article aims to shed light on the nuances that differentiate and unite AI and ML.
Background of AI and Machine Learning
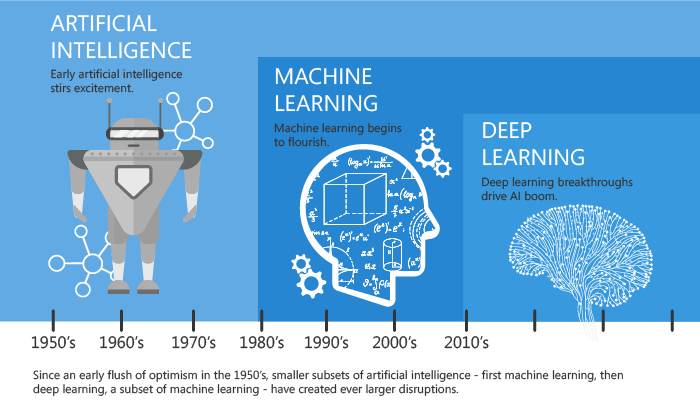
Artificial Intelligence (AI) and Machine Learning (ML) are both facets of the technological revolution that has been reshaping our world for decades. These technologies have roots in computer science, mathematics, and engineering and have been the cornerstone of numerous industry advancements, and hence technology pursuing individuals are increasingly learning from the Artificial Intelligence and Machine Learning courses.
Importance of Distinguishing Between AI and ML
Distinguishing between AI and ML is not just a matter of semantics. It involves understanding the depth and breadth of each domain’s functionalities and applications. This knowledge is vital for academics, industry professionals, and enthusiasts alike to navigate the complex landscape of modern technology.
Brief Overview of the Distinctions and Overlaps
Before we delve deeper, it is crucial to note that while all machine learning is AI, not all AI is machine learning. This statement hints at the broader scope of AI and the specialized focus of ML, a theme that will recur throughout this article.
Understanding Artificial Intelligence
Artificial intelligence defines the imitation of human intelligence in computers designed to think and behave like humans. Any machine that exhibits characteristics of a human mind, such as learning and problem-solving, may also be referred to as AI.
- Historical Background of AI
The concept of AI dates back to the ancient world, but it took a concrete shape in the 20th century with the development of computer science. Over the years, AI has evolved, incorporating complex algorithms and computational power to process information and perform tasks that mimic human intelligence.
The Scope of AI
- General AI
General AI, or Artificial General Intelligence (AGI), defines the machines that possess the ability to understand, learn, and apply their intelligence to diverse domains, much like a human being.
- Narrow AI
In contrast, Narrow AI focuses on performing a specific task or a set of tasks with intelligence without possessing the broad range of human cognitive abilities.
Applications of AI
AI has found applications in numerous fields, including:
- Healthcare: In disease diagnosis, drug discovery, and personalized medicine.
- Finance: For fraud detection, robo-advisors, and automated trading.
- Transportation: In autonomous vehicles and traffic management systems.
- Entertainment: Through recommendation systems and virtual reality experiences.
Various case studies highlight the successful implementation of AI in different sectors, showcasing its versatility and potential to revolutionize industries.
Understanding Machine Learning
Machine Learning, a subset of AI, involves algorithms that improve automatically through experience. It designs and develops computer programs that can access data, learn from it, and utilize it to make business judgments or predictions.
- Historical Background of ML
Machine Learning emerged from the realization that it was not feasible to program computers to perform complex tasks explicitly. Instead, developing algorithms that could learn from and make predictions based on data was more efficient. These concepts are thoroughly understood from the Machine Learning courses, such as Langchain and other alternatives to Langchain.
The Scope of ML
- Supervised Learning
In supervised learning, the machine learning model is trained on a labeled dataset, a set of training examples with associated correct labels. The model makes predictions or decisions based on the input data and is corrected when its predictions are incorrect.
- Unsupervised Learning
Unsupervised learning involves modeling with datasets that don’t have labeled responses. The system tries to learn the underlying patterns and the structure of the data without any supervision.
- Reinforcement Learning
Reinforcement learning is a type of machine learning algorithm based on the concept of agents that take actions in an environment to achieve some notion of cumulative reward.
Applications of ML
Machine Learning has been instrumental in solving complex problems in various domains, including:
- Predictive Analytics: Utilizing data to forecast future events.
- Natural Language Processing: Enabling machines to understand and generate human language.
- Image Recognition: Allowing computers to recognize images and patterns.
Numerous case studies illustrate the successful implementation of ML in different sectors, demonstrating its potential to be a game-changer in the technological landscape.
Conceptual Differences Between Artificial Intelligence and Machine Learning
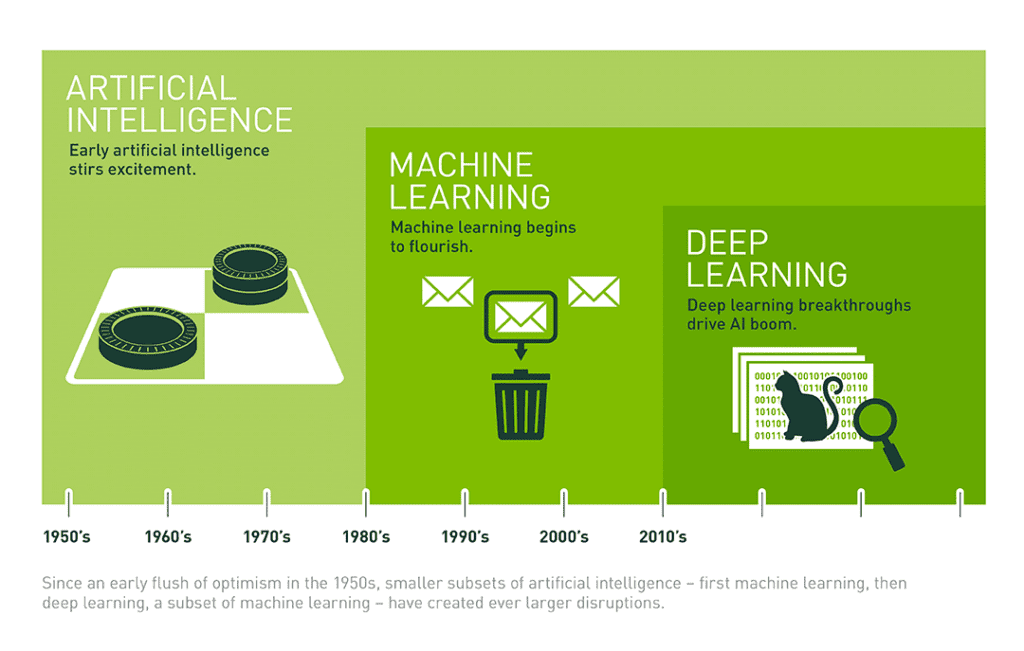
- AI as a Broader Concept
AI is an umbrella term encompassing a wide range of technologies, including machine learning. It represents the broader goal of autonomous machine intelligence.
- ML as a Subset of AI
Machine learning, on the other hand, is a specific approach within the AI domain, focusing on imbibing the ability of machines to learn from data and improve their performance over time.
Technical Differences
- Algorithm Complexity
While AI can involve complex rule-based algorithms, ML relies on statistical and data-driven algorithms to make decisions.
- Data Handling
AI can work with broader data types, while ML specifically focuses on analyzing structured data to make predictions.
- Learning and Adaptability
AI systems may or may not learn and adapt over time, while ML systems are specifically designed and developed to improve their performance as they are exposed to more data.
Practical Implications
- Use Cases
AI and ML find applications in different use cases, with AI focusing on simulating human intelligence and ML emphasizing learning from data.
- Industry Adoption
Different industries adopt AI and ML technologies based on their specific needs and the problems they are trying to solve.
Overlaps Between AI and ML
Shared Goals
- Automation
Both AI and ML aim to automate tasks that would otherwise require human intervention, thereby increasing efficiency and accuracy.
- Data Analysis
AI and ML share the goal of analyzing data to discover valuable insights and make informed decisions.
- Predictive Modeling
Both domains utilize predictive modeling to forecast future events based on historical data.
Complementary Technologies
- Deep Learning
Deep learning serves as a bridge between AI and ML, utilizing neural networks to analyze various forms of data.
- Neural Networks
Neural networks form the backbone of many AI and ML applications, simulating the human brain’s interconnected neuron structure to process data.
Collaborative Applications
- Voice Recognition
Voice recognition technologies often utilize both AI and ML to understand and process human speech.
- Autonomous Vehicles
Autonomous vehicles are a prime example of the collaboration between AI and ML, utilizing various technologies to navigate and operate without human intervention.
Several case studies highlight the successful collaborative implementations of AI and ML in different sectors, showcasing their synergistic potential.
Conclusion
As we look towards the future, we can be sure to see continued growth and evolution in both the AI and ML domains, with new trends emerging and shaping the technological landscape. Potential developments in AI and ML could revolutionize industries, bringing about unprecedented changes and advancements. Understanding the distinctions and overlaps between AI and ML is crucial for fully leveraging their potential and fostering innovation in various fields. As we navigate the complex world of AI and ML, it becomes increasingly important to understand their nuances. This knowledge can be a foundation for further exploration and innovation in these exciting domains.
Check out more AI tool.
Elevate Guest Experience with RoomGenie
🚀 Check out NewsGenie – Your AI consultant