More and more businesses across a wide range of industries are implementing AI to improve operational efficiency, automate repetitive tasks, and gain competitive advantage on the market. Yet, despite the growing interest, 85% of AI projects fail, according to a report published by Gartner. This high failure rate points to companies facing difficulties with AI implementation. In this article, we explore the main reasons behind that and explain how companies can prevent AI project failure. By addressing common considerations head-on, businesses can better navigate the complexities of AI projects and increase the chances of positive outcomes.
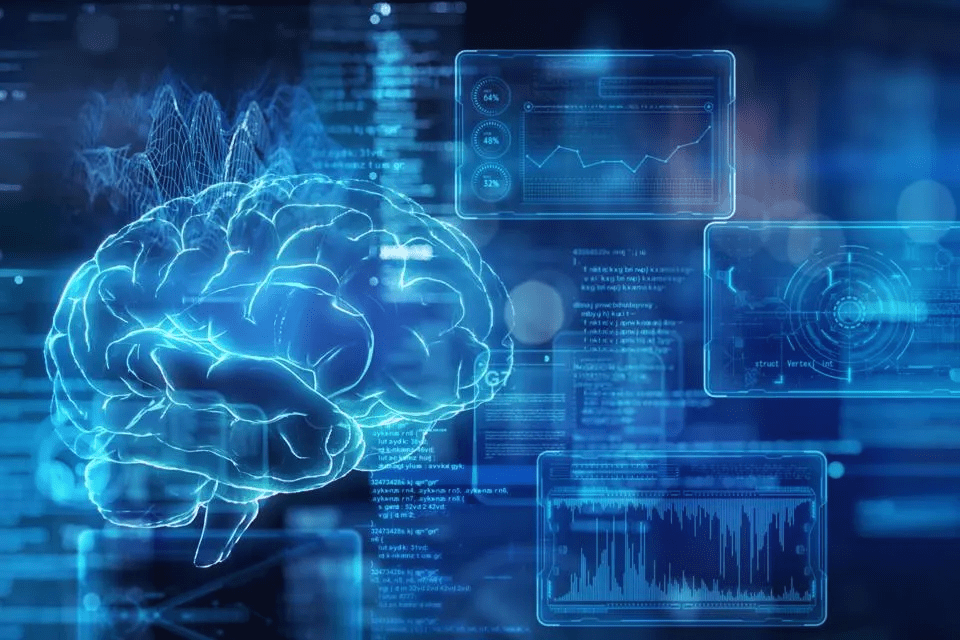
A clear business case is key
A clear business case is essential. Poor project scope and misunderstanding AI tasks can lead to unmet expectations and little value. Start by preparing a detailed business case. This involves evaluating ideas, identifying problems, and defining objectives. After these steps, check business and tech feasibility. Ensure the AI project matches your capabilities. Consider which technology fits your goals. The right idea and technology combination maximizes ROI and aligns AI with strategic goals.
Data needs to be thoroughly prepared
Data is at the core of AI solutions and machine learning models require vast amounts of data to learn patterns and make accurate predictions. Before initiating AI implementation, businesses need to make sure they have enough data to feed and train algorithms. Using insufficient data can result in AI models underperforming or not delivering meaningful insights.
However, the volume of data is not all that matters. Datasets we use must be clean, properly annotated, and closely aligned with the specific tasks our AI model is intended to perform. Just like not having enough data, its poor quality can lead to incorrect model predictions, ultimately affecting the effectiveness of our AI solution. The data preparation process can be complex but is essential for success. Additionally, it is important to continually update and refine datasets to maintain their relevance as conditions and requirements change.
The project must be within our capabilities
According to Johan Himberg and Ville Koistinen, many organizations fail because they overestimate what can be achieved with their current capabilities. Before diving into an AI project, it is essential to assess our technological infrastructure. This involves a thorough evaluation of what resources we currently have and identifying any gaps that need to be filled. Choosing the right technology stack is essential for seamless integration and long-term success. But what is a technology stack? It is the combination of programming languages, tools, and frameworks used to build and run AI applications. A well-chosen technology stack can make or break an AI project, as it dictates the ease with which a system can be maintained, scaled, and integrated into existing processes.
Moreover, the stack should be aligned with the team’s skill set, ensuring that those who will be managing the AI solution are familiar with the tools and frameworks being used. It is important to note that sometimes investment into new tools might be necessary as the field of AI is constantly evolving and new frameworks become available.
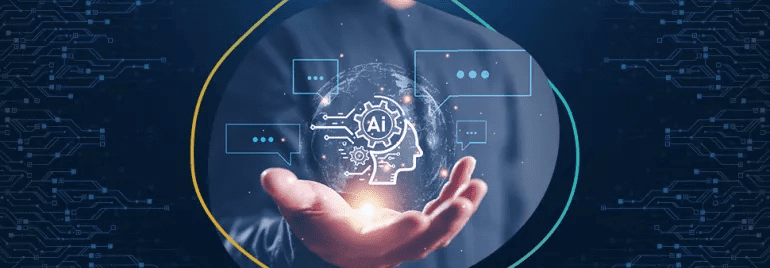
The right team matters
Once we determine that we have the required data and technology, we also need the talent to make our vision come true. And unfortunately this often turns out not to be an easy task. A good AI team should gather data scientists, machine learning engineers, domain experts, software developers, data engineers, and business analysts. Each of these roles plays a vital part in the AI project lifecycle, from initial conception to deployment and ongoing maintenance. For example, data scientists and machine learning engineers develop the algorithms that power AI systems, while software developers and data engineers take care of the integration of AI into existing systems, ensuring data integrity and continuity of business operations. Domain experts, on the other hand, provide industry-specific insights, and business analysts translate business objectives into technical requirements.
Businesses that start AI projects without experienced AI experts onboard risk significant setbacks and may find themselves unable to deploy effective AI solutions. A lack of skilled personnel can lead to poorly designed models, inefficient workflows, and eventually, failed AI projects.
Ethical considerations must be considered
As AI adoption in business is on the rise, concerns revolve around fairness, accountability, transparency, and privacy. Bias in AI models is a potential risk that can result in unfair treatment of individuals or groups, deepening existing inequalities. Why does this happen? Such a problem can occur when AI models are trained on biased datasets, reflecting historical prejudices present in the data. Luckily, businesses are capable of mitigating this risk by conducting thorough audits of their datasets and models. Implementing bias detection and correction methods can help ensure that AI systems are designed and deployed responsibly. Furthermore, AI projects must adhere to legal and regulatory frameworks that govern data usage, privacy, and algorithmic accountability. In the European Union the main piece of legislation that addresses these issues is the General Data Protection Regulation (GDPR).
Summing up the keys to AI project success
The high failure rate of AI projects highlights the need for a strategic approach. Start with a clear business case and prepare your data properly. Align your technological capabilities and assemble a skilled team. Follow the best practices discussed in this article to avoid common pitfalls. By doing so, businesses can greatly increase the chances of AI project success. A holistic approach not only improves technical and operational aspects but also ensures responsible deployment of AI solutions.
Check out more AI tool.
Elevate Guest Experience with RoomGenie
🚀 Check out NewsGenie – Your AI consultant